Monte Carlo Roulette 26 Times
Monte Carlo is famous for its casinos. Many of the activities presented therein offer (supposedly) random outcomes. For example, you might bet on red or black on the roulette wheel. Some of the events that influence investment returns are also seemingly random. For example, whether a tenant exercises a break option or leaves at the end of its lease may well be something that you can not influence directly. Indeed it may be a totally random decision as far as the investor is concerned. Nonetheless, we might have some idea as to the likelihood of a decision to leave, based upon historic information, expectations about the economy and possibly knowledge of the tenant itself.
Monte Carlo simulation assumes a random outcome for each individual event. If this hypothesis is run many times, we can see a distribution of the resulting returns that results from the combination of these events This informs us about the likelihood of hitting a defined hurdle rate, the most likely return level and the nature of the possible spread of returns (and so our risk).
Our implementation of this methodology might be likened to a deck of cards. If the deck is shuffled and each time a binary event (such as a tenant exercising a break option) is reached, a card is drawn at random from the deck. If the card is red the tenant stays, if it is black it leaves.
Given that we have some information about the likelihood of the tenant staying or going, we can stack the odds. This is done by simply changing the ratio of red to black cards in the deck. For example, if we expect a c. 30% likelihood (or probability) of the tenant leaving, we could remove 16 black cards. The result would be that there are 26 red cards and only 10 black cards. We therefore have a 27.8% chance of picking a black card (52 /2 = 26 cards of each colour. Remove 16 black and we have 10 black cards left, 8/36 = 0.278).
Monte Carlo Roulette 26 Times 3.14
In 1913, gamblers at the Monte Carlo Casino lost millions of francs at the roulette table. The ball would land on black, and the gamblers would bet on red. The ball would land on black again, and the gamblers would continue to bet on red. The ball landed on black 26 times in a row. By Laura Snider. During a famous roulette game in a Monte Carlo casino in 1913, black came up 26 times in a row. After about 15 repetitions, the players began betting heavily on red, likely believing that such a long streak just couldn’t continue. In August 1913, a game of roulette at a casino in Monte Carlo attracted the attention of a crowd of gamblers when the ball landed on black numbers 26 times in a row. The longer this streak of black numbers continued, the longer the game seemed to play up to the fallacy: surely, the gamblers started to feel, a red number was now overdue?
Of course the application does not use playing cards and it does not limit itself to 52 cards. Instead it creates a list of 100 0s and 1s in the proportion of the probability inputted by the user. It then makes a random choice each time a binary lease event is reached. This gives us one possible outcome. The application then repeats this process many times in order to reach a distribution of expected outcomes, shown as a histogram.
In this way the application is able to offer some insight about a) the most likely result in terms of financial performance, b) the expected spread of results and c) the skew of these results towards the positive or the negative.
Using this information, applied to a set of assumptions based upon expected worst case, it is possible to estimate the Value at Risk (VAR), ie. the amount of loss incurred for a given number of standard deviations from the mean return.
There are several different ways of measuring the risk of a financial investment. Historic volatility is a commonly used metric. For real estate, we often consider more subjective factors, such as the quality of location, the flexibility of the building, the covenant strength of the tenants etc.
A commonly used approach to risk in other sectors is known as VAR or Value at Risk. VAR methodology aims to determine the potential for loss of the capital invested under a 'defined' probability set. For example, we could look at historic market movements and how often they occur and then use this data to project the likelihood of loss. Alternatively, we could look at data from previous downturns or market shocks and apply them to our model. We can add this to data from the Monte Carlo analysis described above (which will add in the probability of 'random' events). In this way it is possible to construct a 'credible worst case scenario'. By looking at capital lost in the scenario we can gain some insight as to how risky a project is; by effectively measuring the expected maximum losses for a given probability/certainty level.
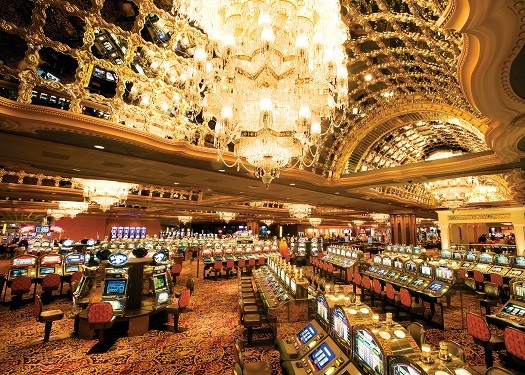
Multi-factor risk scoring provides a framework within which to think about the risk of a particular investment. It is somewhat of a subjective exercise, and although it does produce a result (an investment hurdle), this should be thought of as a guide only. The process itself of thinking about the various contributors to the risk of the project is at least as important as the result.
In 1913, gamblers at the Monte Carlo Casino lost millions of francs at the roulette table. The ball would land on black, and the gamblers would bet on red. The ball would land on black again, and the gamblers would continue to bet on red.
The ball landed on black 26 times in a row. The probability of that happening was 1 in 66.6 million. To this day, it’s a shocking moment in gambling history.
The 27th time, can you guess what the probability of the ball landing on black would be?
1 in 2, or 50/50.
This doesn’t seem right, but it is. And the people who would have bet on black, after the ball landing on black 26 times in a row, would still only win double what they bet.
This video is all about the Gambler’s Fallacy, and how we convince ourselves that things will “even out” on the gambling table and in other change occurrences.
What Is The Gambler’s Fallacy?
Put yourself in the gamblers at Monte Carlo’s shoes. The ball lands on black. The ball lands on black again. The ball lands on black for a third, fourth, fifth time.
At some point, you’re going to feel sure that the ball will land on red next. There’s a 50/50 chance that the ball will land on red at any given time or on black at any given time. So after a streak of the ball landing on black, you may start to think that the ball has to land on red next.
This is the gambler’s fallacy. It’s a bias in which we let past events influence our decisions and predictions about what will happen next. But this bias is based on fallacy, or a mistaken belief.
The reality of the situation is that the ball has a 50/50 chance of landing on black every single time you play roulette. Each action is independent of the actions before it. Sure, the chances of the ball landing on black 26 times in a row are way smaller than the chances of the ball landing on black twice or three times in a row, but that doesn’t mean that the ball will land on red based on those chances.
The gambler’s fallacy often leads people astray while they’re in the casino. Think of all of the people who continued to bet on red at Monte Carlo. I’m sure some of those gamblers put a lot of money on red, thinking that somehow their chances at winning big were larger just because the ball kept landing on black.
But this doesn’t just happen at the roulette table. Many people will stay at the slots for hours, believing that although they lost time after time again, their chances of winning will somehow increase.
Examples
Monte Carlo Roulette 26 Times
But the gambler’s fallacy isn’t just a phenomenon that occurs within the walls of a casino. In the “real world,” this logical fallacy can have some pretty serious effects on the ways people make decisions and conduct business.
Take loans. Approving or rejecting loans can sometimes feel like a gamble to loan officers. Studies show that loan officers are more likely to reject a loan that is sitting in front of them if they had approved the last loan that came across their desk. Researchers think that 5% of loans are affected by this fallacy.
This can have a huge impact on a person’s ability to buy a house or start a business, but further research shows that this fallacy can have an even more significant impact. Asylum judges are not immune to the gambler’s fallacy. Data shows that they are more likely to approve a person’s application if they had denied the previous application, and vice versa.
Explanations

The gambler’s fallacy goes beyond how we make decisions - some argue that it affects how we make sense of the world. Remember, the gambler’s fallacy is a bias that is influenced by past events. We may link our decision to stay at the slots because of past events. But we may also explain what is happening now based on what happened in the past.
We use the gambler’s fallacy to establish patterns. We may get sick and blame it on the bread that we ate before. If we get sick a second time after eating bread, we are very likely to believe that we have an intolerance or allergy involving gluten. But connecting our stomachache to what we ate before isn’t always based in logic or correct probability. Just like connecting the ball landing on red to the ball landing on black before (or vice versa) isn’t always based in logic. Independent events are just that - independent events.
Be Aware of Gambler’s Fallacy
I’m not saying that people with stomach aches shouldn’t write off certain foods or that you should go to the casino and only bet on one color or number. But I am telling you about the gambler’s fallacy so you can be more aware of if you’re using it in your life.
Logical fallacies affect the way we make decisions, form arguments, and predict what is going to happen in the future. Since these fallacies are based on falsehoods, the results may not be so great. The more you are aware of these logical fallacies, the easier it will be for you to step back, make a proper assessment based on facts, and make better sense of what is going on around you.
Quiz
Let’s test your knowledge on the Gambler’s Fallacy.
Question 1:
Monte Carlo Roulette
A coin has been flipped 12 times. 10 of those flips have resulted in “heads.” What is the likelihood that it’s going to be tails the next time you flip it?
Answer:
50/50
Question 2:
True or False: The Gambler’s Fallacy is just limited to gamblers.
Answer:
Monte Carlo Roulette 26 Times 16
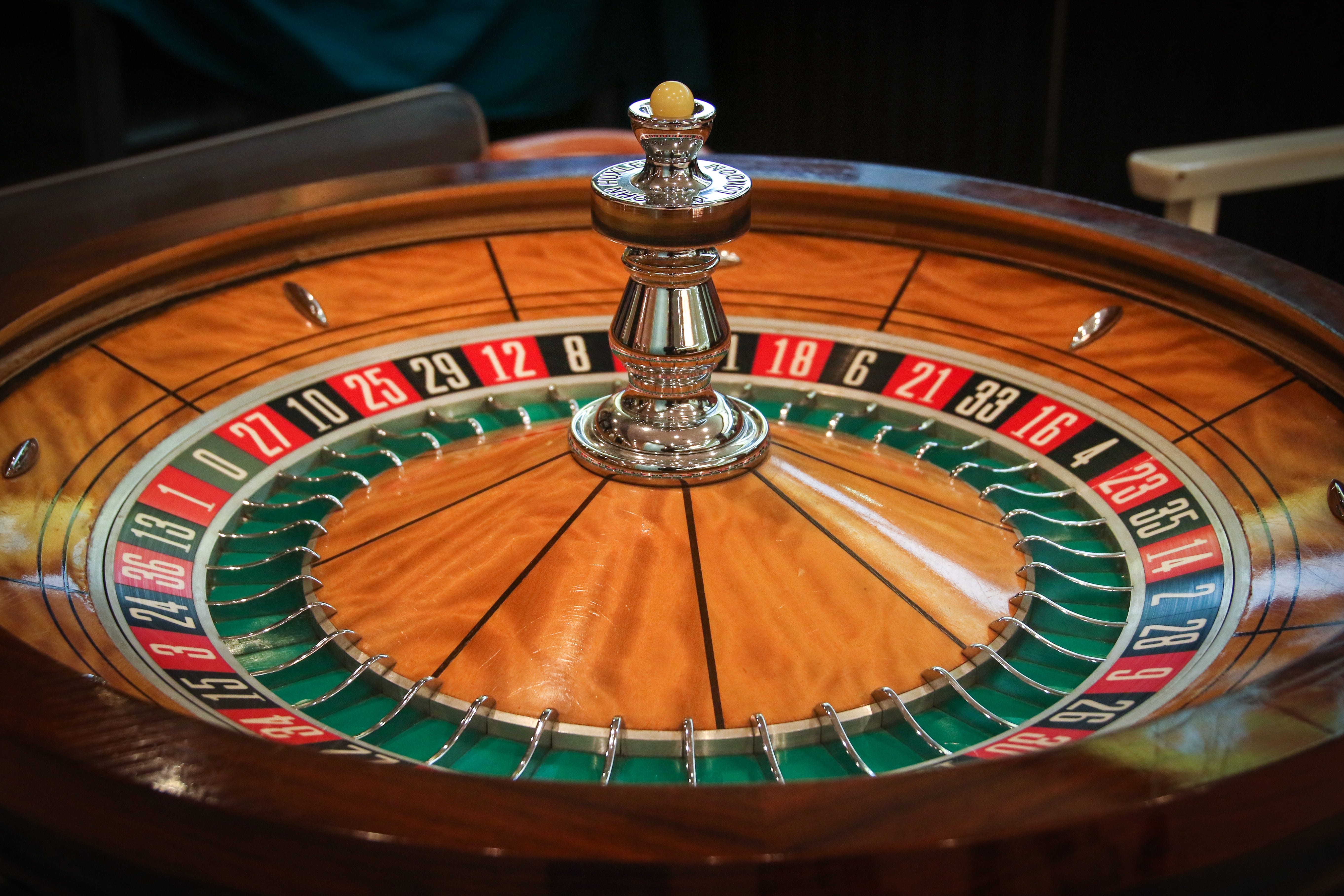
False. Everyone is affected at some point by the Gambler’s Fallacy. It’s a common bias that all humans experience.
Last question:
The Gambler’s Fallacy is a _____ fallacy that we should be aware of when making judgements and decisions.
Answer:
Logical